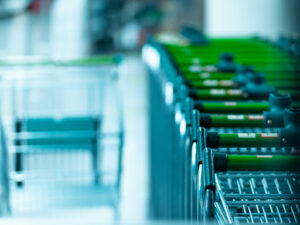
GenAI is a game changer for supply chain planning as it takes planning closer to being autonomous…
Demand planners work hard with seemingly little reward or recognition. This should be no surprise – if your forecast accuracy is good and activities across the supply chain work well to deliver target outcomes, you did your job. If your forecast accuracy is low or worse, you failed.Â
Often, forecast accuracy, even of median performers, is mediocre, particularly at a practical level – SKU or product – versus product family or other aggregated levels. But it no longer has to be this way. Harnessing the strides in genAI capabilities since 2022 has made it easier to transform demand planning.
Why This Matters
Even without undertaking a broader supply chain planning transformation, improving forecast accuracy can have a significant impact on the business. Further, improving demand planning is foundational to advancing other planning capabilities – inventory management, supply planning, and sales & operations planning.
Based on our experience, approximate improvements possible by moving from median to top quartile performance just by improving demand planning is financially rewarding and operationally compelling. The approximate improvements are shown in exhibit 1. The substantial reduction in planning effort is based on genAI and semi-autonomous planning enablement.
The improvements are demonstrably higher when all supply chain planning capabilities are addressed. However, the related planning capabilities are not the focus of this article.
Zoom Out
GenAI can help make a step-change improvement in overall supply chain planning, and demand planning is the foundational starting point. GenAI can integrate structured and unstructured data from historical demand and customer forecasts to customer sentiment and market activity. The improvement in forecast accuracy with genAI can be 30 pp or higher. A median performer can reach today’s best-in-class level. And if you’re best-in-class, you can still make meaningful improvements as supply chains get more complex, consumer preferences more volatile, and their expectations rise.
What makes such a dramatic improvement possible versus previous conventional techniques are:
A rapid and iterative approach helps get started:
1. Start with a pilot, e.g., a product line, a geo, or a customer segment.
Commercially available genAI platforms and models are sufficient for rapid prototypes and pilots.
2. Deploy, learn, and iterate rapidly to improve outcomes continuously
Operate in parallel with your current tools and process to measure improvements and correct issues.
Establish thresholds of performance for metrics such as accuracy, bias, and volatility.
3. Industrialize and expand
After two to three cycles, consider industrializing the solution to expand scope. This requires higher investment in technology and implementation but the business case should be compelling based on pilot results.
4. Set the demand planning process on auto-pilot and let it run, monitor, and fine-tune
Operate the new demand planning engine and monitor deviations by establishing suitable thresholds for human intervention and improvement.Â
Zoom In
Looking at demand planning as an intelligence synthesizing effort versus a data manipulation process is a paradigm shift. Specifically, there are three categories of intelligence gathered and synthesized:Â
Exhibit 2 shows how to implement the Consensus Planning process – synthesize information, integrate the planning steps, and incorporate human intelligence.
Implementation Steps
The cycle time for the entire process is an order of magnitude less than any current planning cycle time because of the speed, intelligence, and interactivity of technology.
Watch-out
Despite the intelligent capabilities of genAI and other advanced planning applications, there is no substitute for expert-led human leadership. In our experience, this is where companies struggle. Abdicating human accountability for the quality of forecasts is a recipe for mediocrity at best, failure at worst.
Call to Action
If you have not yet implemented genAI in your demand planning process, you are nearly two years late, and it is time to catch up. Consider doing it now. Pilots can be done in as little as two to three weeks. And successful pilots can be industrialized in one to two 90-day sprints.
You can extend this to other areas of supply chain planning (e.g., supply planning, inventory management, S&OP) in a phased manner to drive an overall autonomous supply chain planning. CI
Analysis Note
Our modeling and analysis with CASETEAM’s Prism suite have demonstrated the transformative impact of using genAI on demand planners’ workflows, achieving an estimated 90% reduction in effort.
Tasks like aggregating data, analyzing trends, and producing high-quality forecasts—traditionally time-intensive and error-prone—are now streamlined with genAI, allowing planners to focus on strategic decision-making rather than data gathering, synthesis, and administrative burdens. This substantial efficiency gain enables demand planners to deliver more accurate, actionable insights faster, positioning the supply chain function to respond swiftly to market dynamics.
By integrating genAI, demand planning transforms from a labor-intensive, moderate accuracy effort to a high-impact, strategic function.
The $24.6 billion Kroger-Albertsons merger, announced in 2022, aimed to create a grocery powerhouse to compete with Walmart and Amazon. However, regulatory opposition and public backlash…
Mergers and acquisitions are among the most transformative moves a company can make to accelerate growth — but it is commonly known that 70% or more of M&A transactions fail …
In today’s highly interconnected economy, supply chain resilience is no longer just a defensive strategy…
Between the forces of genAI disrupting all industries and a change of administration in the US, there is a widespread belief that M&A activity will pick up.
By Srini Bangalore, Founder & Chief Client Officer | Cate, genAlyst
CASETEAM Launches as GenAI-native and Hybrid-first Management Consulting Firm, Innovating a New Model that Makes Top-tier Problem-solving Accessible to More Organizations
By Integrating GenAI with Human Ingenuity, CASETEAM Creates a New Model for Management Consulting
Q&A with Cate, genAlyst at CASETEAM
[Note: Cate is a genAI model configured for the needs of CaseTeam]
Share this Post